Imagine if the greatest technological inventions in history—such as the steam engine, electricity, or the Internet—had been left stranded in research laboratories and never transferred to society. The world would look radically different today. There are plenty of untold stories of inventions that never saw the light of day, and many potentially breakthrough ideas fall into the so-called technological “valley of death” due to a gap between academic research and industrial commercialization. This is a missed opportunity for economic and social progress. In this article, I offer five actions for academia and industry to bridge the valley of death and co-create innovation.
In recent years, the public has often associated technological innovation with the most successful private companies in the world, such as Apple, Google, and Microsoft. These organizations have built an entrepreneurial culture characterized by networks (rather than hierarchies), empowerment (rather than control), and agile execution (rather than rigid planning). While these factors play a role in fostering technological innovation, one of the fundamental drivers remains technology research. Unlike traditional research—which aims at obtaining new knowledge about the real world—technology research aims at producing new and better solutions to practical problems.
Consider the disruption brought to the smartphone market by the iPhone. The user experience of Apple’s first smartphone was undoubtedly superior to the competition, and this was probably the recipe for its success. However, a significant portion of the technologies leveraged by the iPhone was originally conceived in research conducted by the public sector. The programming languages used in the iPhone, for instance, have roots in the research conducted between the 1950s and the 1980s by multiple organizations worldwide, including the Massachusetts Institute of Technology and the Norwegian Computing Center.
The journey of new technology from research to commercialization goes through a number of technology readiness levels (TRLs). These levels were developed at NASA between the 1970s and the 1990s, and indicate the maturity of technologies. The latest version of the scale from NASA includes nine TRLs and has gained widespread acceptance across governments, academia, and industry. The European Commission adopted this scale in its Horizon 2020 program.
The nine technology readiness levels are:
- Basic principles observed
- Technology concept formulated
- Experimental proof of concept
- Technology validated in a lab
- Technology validated in a relevant environment
- Technology demonstrated in a relevant environment
- System prototype demonstrated in an operational environment
- System complete and qualified
- System deployed in an operational environment
Some tech giants are large enough to fund research units and work at all levels of this scale, but most companies cannot afford the high investments and specialized competencies this approach requires. Either way, in today’s dynamic and volatile markets they have to aim at doing the disrupting in order to avoid being disrupted. Companies that cannot afford research units have to innovate by relying on research conducted elsewhere, and the natural candidate is academia.
The question is: at which TRL does academia transfer technology to industry?
Academia tends to focus on TRLs 1–4, whereas industry prefers to work with TRLs 7–9, rarely 6. Therefore, TRLs 4–6 represent a gap between academic research and industrial commercialization. This gap is colloquially referred to as the technological “valley of death” to emphasize that many new technologies reach TRLs 4–6 and die there.
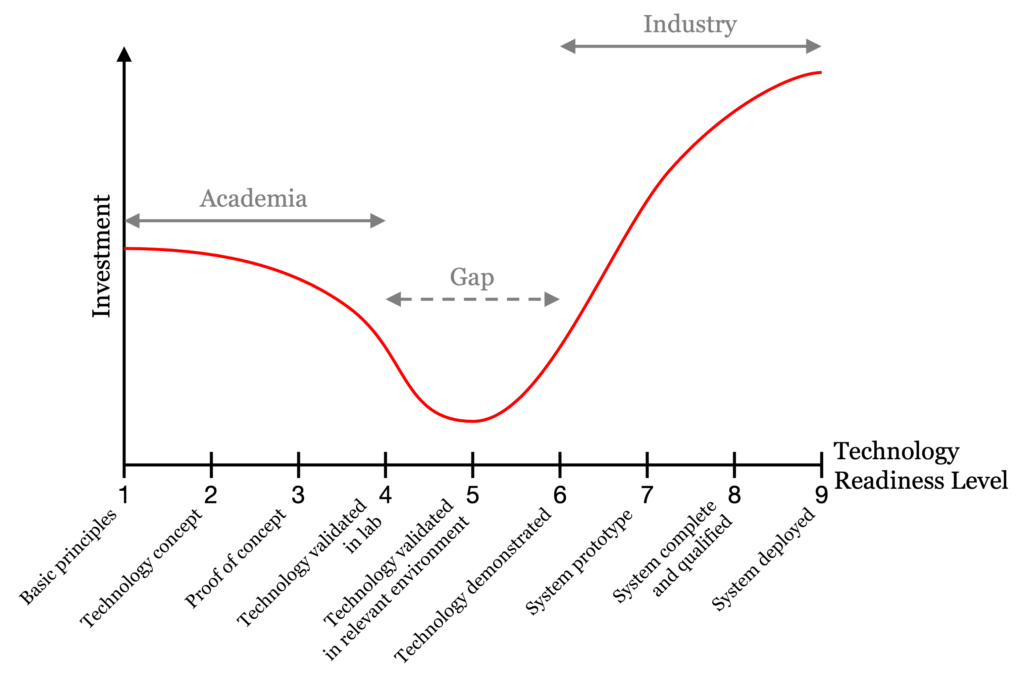
The issue of the valley of death has been studied extensively, and the scientific literature offers several proposals for bridging the gap. With the aim of facilitating technology transfer, some research organizations have focused entirely on technology research in the last 50 years. The Fraunhofer Society in Germany and SINTEF in Norway are notable examples. Still, these efforts have not entirely bridged the gap between academia and industry.
So, how can academia and industry bridge the technological valley of death and co-create innovation?
1. Academia and industry should better understand each other’s culture
To paraphrase a well-known proverb: academia is from Mars and industry is from Venus. This is not only reflected in the way people work but also the way people communicate and possibly dress. The following statements are deliberate exaggerations, but they allow pondering on the cultural differences in the status quo. Academics have a long-term horizon; practitioners have a short-term horizon. Academics think (often critically); practitioners do (often routinely). Academics are pedantic; practitioners are practical. And the list could go on and on… Note that academia is not superior to industry, and industry is not superior to academia. They are just different, and this diversity can be healthy to drive technological innovation. Understanding each other’s culture is of paramount importance to improve the collaboration across teams on both sides.
2. Academics should better understand real-world industrial challenges
Academic research tends to aim at academic communities. As a consequence, an increasing number of research papers do not have adequate industrial relevance. As Lionel Briand—a recognized professor and researcher in software engineering—recently put it: “If a solution to a problem is not applicable and scalable, at least in some identifiable context, then the problem is not solved. It does not matter how well written and sound the published articles are, and how many awards they received.” Academics should join industrial fora (conferences, seminars, etc.) more often in order to understand real-world industrial challenges.
3. Practitioners should stay up-to-date with the state-of-the-art
The fact that certain research papers do not have an adequate industrial relevance does not mean that industry cannot resort to the scientific literature to find answers to its questions. Quite the contrary: the answer is often available in peer-reviewed journals, many of which are openly accessible. Consider, for instance, password expiration policies. There is evidence that changing passwords on a regular basis does more harm than good since it drives users to new passwords that can be predicted based on previous passwords. Nevertheless, most IT departments keep enforcing this policy rather than adopting modern authentication solutions. Imagine if medical doctors would neglect research results in the same way… Unrealistic, right? Practitioners should join academic fora and consult the scientific literature more often in order to stay up-to-date with the state-of-the-art.
4. Industry should hire more PhDs
Besides the qualities that may make PhDs more valuable than other job candidates, people who invested at least three years of their lives researching in academia know the environment well enough to foster: the identification of research trends, the understanding of research results, and—most of all—the collaboration with academia. In the process of academic inflation given by a steadily increasing number of doctorates every year, the academic pyramid is getting wider, and not all PhDs have the opportunity to access permanent academic jobs. Industry has the chance to capitalize on this trend by hiring more PhDs.
5. Academia and industry should conduct more joint research projects
Academia and industry can organize their joint research projects based on two models: 1) bilateral collaboration, where both academia and industry provide their contribution in the form of cash or in-kind, or 2) research projects partly funded by governmental organizations. The latter option may be better suited in case of tight budgets and lack of experience with this kind of collaboration.
Research is by definition unpredictable, and research projects cannot be assessed based on cost-benefit analysis. Nevertheless, statistics on past research projects show high long-term return on investment. The European Commission and the Norwegian Research Council (as well as other governmental organizations around the world) have a wide variety of research programs in a heterogeneous set of domains, which should be sufficient to cover the needs of most establishments. The competition for accessing funding has increased dramatically in the last decade, so the stakeholders should pick their partners and prepare their funding applications carefully.
While the success stories of fruitful collaboration between academia and industry are encouraging, there is a lot of untapped potential in the synergy between the two. Understanding each other’s culture, joining each other’s fora, leveraging PhDs’ competence and skills, and conducting joint research projects are certainly steps in the right direction. Technology has been responsible for tremendous economic growth and increased quality of life for billions of people. Bridging the technological valley of death is definitely worth solving, regardless of whether the ultimate goal is an increased profit or the greater good.
A shorter version of this article was published in Norwegian in Teknisk Ukeblad on February 2019.